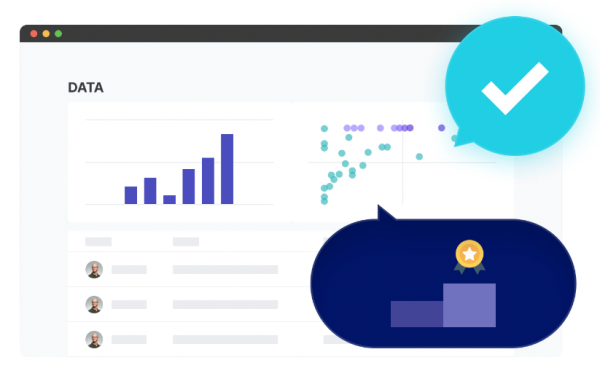
Have you heard of data analysis? Data analysis plays a crucial role in modern scientific and technological research, especially in STEM (Science, Technology, Engineering, and Mathematics) fields, where it provides strong evidence and insights. Let's explore why data analysis is essential when conducting research in STEM fields.
The Importance of Data Analysis in STEM Research What is Data Analysis? So, what exactly is data analysis? Simply put, data analysis involves examining and understanding collected information or data to derive useful insights. It includes analyzing data using various methods to find patterns, rules, trends, and relationships that help in decision-making and problem-solving. Data analysis helps uncover hidden information within complex data, predict future trends, and establish improved decisions and strategies.
The Process of Data Analysis 1. Data Collection Gather information or data of interest. This data can come from experimental results, surveys, sensor data, user behavior on websites, and more. 2. Data Cleaning Clean and refine the collected data to remove unnecessary information and errors. Incomplete or inconsistent data can distort analysis results, making this a critical step. 3. Data Transformation Transform or summarize the data to make it easier to analyze. For instance, summarizing sales data by month or calculating averages over a specific period. 4. Data Exploration Explore the characteristics and patterns in the data. Use visualization tools to create graphs or charts, and identify distributions, relationships, and outliers. 5. Statistical Analysis
Use mathematical and statistical techniques to identify meaningful patterns or relationships in the data. This can involve building predictive models or testing hypotheses. 6. Modeling and Prediction Develop mathematical models from the data or apply existing models to predict future events or outcomes. 7. Result Interpretation Understand and interpret the analysis results to derive insights that can be used in decision-making. 8. Decision Making and Application Use the analysis results to solve problems or develop strategies.
Advantages of Data Analysis With the rise of big data, the field of data analysis and data science has become increasingly significant. Data analysis and data science are becoming less distinguishable, especially since data is crucial in AI. Handling data is an essential skill across all STEM fields.
Data analysis helps in: - Identifying patterns and relationships - Developing decisions and strategies - Deriving new insights
Skills Required for Data Analysis 1. Ability to Develop the Question The first essential skill is the ability to develop the problem. Users often struggle to articulate their problems appropriately. Therefore, data scientists must frequently collaborate with business experts to identify the issue. 2. Ability to Question the Data Experienced professionals do not just run raw data through analysis programs and accept the results. They need to filter and adjust data characteristics carefully to avoid misleading conclusions.
Why is Data Analysis Important in STEM? 1. Provides a Basis for Evidence and Judgment Data analysis is used to verify theories or review hypotheses by analyzing various forms of information, such as experimental results, observational data, and model outputs. This allows scientists to derive new understandings and explanations of phenomena. 2. Enables Accurate Conclusions By collecting and processing information through data analysis, potential misunderstandings or incorrect conclusions can be avoided. Statistical techniques can also be used to assess the reliability and significance of results, making conclusions more robust. 3. Model Development and Improvement Data analysis is essential for developing and validating models. Scientists use data to create mathematical models explaining phenomena and adjust these models for more accurate predictions.
Examples of Data Analysis in STEM Ex 1. Chemistry Data analysis is used to study molecular structures, chemical reactions, and the properties of chemical substances. It helps in understanding and explaining chemical phenomena through spectral analysis, mass spectrometry, and molecular modeling. Ex 2. Materials Research Data analysis aids in understanding and calculating the characteristics and performance of various materials. It helps in studying the strength, durability, and electrical conductivity of materials by analyzing experimental results to find optimal material compositions.
Data Analysis in AP Programs Data analysis is not only significant in STEM fields but also in various areas in modern society, which is why it's heavily covered in high school AP programs. 1. AP Statistics
Focuses on data collection, analysis, and interpretation. The course covers methods for data collection, descriptive statistics, probability, and inference, providing a foundation for analyzing and interpreting data in real-life situations. 2. AP Computer Science A Covers the basics of programming and computer science but also includes aspects of data processing. Topics related to data structures, algorithms, databases, and data manipulation are connected to data analysis.
The Importance of Data Analysis for In-Depth Research So, is data analysis essential for college admissions to prestigious universities in the U.S., given its emphasis in various AP courses? The answer is "yes." While this article focuses on examples from STEM fields, data analysis is crucial across all disciplines. Students with data analysis skills are considered to have advantages such as: - Effectively utilizing information - Possessing highly demanded technical skills - Solving complex problems In conclusion, mastering data analysis is critical for conducting comprehensive research and succeeding in various academic and professional fields. |